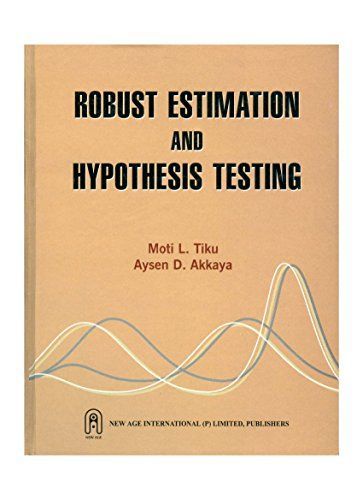
Robust Estimation and Hypothesis Testing
In Statistical Theory And Practice, A Certain Distribution Is Usually Assumed And Then Optimal Solutions Sought. Since Deviations From An Assumed Distribution Are Very Common, One Cannot Feel Comfortable With Assuming A Particular Distribution And Believing It To Be Exactly Correct. That Brings The Robustness Issue In Focus. In This Book, We Have Given Statistical Procedures Which Are Robust To Plausible Deviations From An Assumed Mode. The Method Of Modified Maximum Likelihood Estimation Is Used In Formulating These Procedures. The Modified Maximum Likelihood Estimators Are Explicit Functions Of Sample Observations And Are Easy To Compute. They Are Asymptotically Fully Efficient And Are As Efficient As The Maximum Likelihood Estimators For Small Sample Sizes. The Maximum Likelihood Estimators Have Computational Problems And Are, Therefore, Elusive. A Broad Range Of Topics Are Covered In This Book. Solutions Are Given Which Are Easy To Implement And Are Efficient. The Solutions Are Also Robust To Data Anomalies: Outliers, Inliers, Mixtures And Data Contaminations. Numerous Real Life Applications Of The Methodology Are Given.