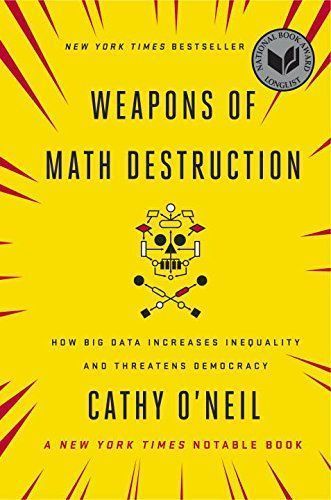
Reviews
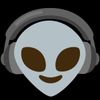
Weapons of Math Destruction is a quick read about the social impacts of mathematical modeling. O'Neil's writing is engaging and you don't really have to know anything about math/statistics to understand it. With accessibility there's a loss of detail, though. It's all very surface level, and most of the case studies will probably be familiar to you if you have read tech news with any regularity within the last five years. At one point O'Neil quips that political speeches are often boring because they're trying to be appealing to basically everyone, and that is how I feel about this book. O'Neil shies away from the logical conclusion (critique of capitalism, because capitalism necessitates the sort of inequalities driven by WMDs that O'Neil calls out in the text). The conclusions she does offer up aren't all that well-formed or compelling.
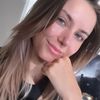
Eye opening book. A must read if you’re interested in the world of privacy and big data.
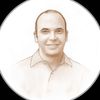
Nice ideas in an easy-to-read format.
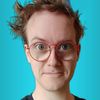
Important and flawed. It is very hard to think clearly about these things (witness the many inconsistent uses of the term "bias" in the field) but O'Neil goes some way toward this. She is more balanced than average, recognising that algorithms can be an improvement over human bias and pettiness (she praises FICO scores as the liberating thing it was, moving money from those bank managers liked to reliable people of any stripe). The mass, covert automation of business logic in schools, universities, policing, sentencing, recruitment, health, voting... Much to admire: she is a quant (an expert high-stakes modeller) herself, understands the deep potential of modelling, and prefaces her negative examples with examples of great models, methods of math construction. She was one of the few technically literate people in Occupy: in mid-2011, when Occupy Wall Street sprang to life in Lower Manhattan, I saw that we had work to do among the broader public. Thousands had gathered to demand economic justice and accountability. And yet when I heard interviews with the Occupiers, they often seemed ignorant of basic issues related to finance... They were lucky to have her! Following recent convention, she calls the decision systems 'algorithms'. But it isn't the abstract program that does the harm, but being credulous about their predictions. Programs only do harm when they are allowed to make or guide decisions. She flip-flops between thinking that false positives are the problem, and that any positives based on uncomfortable variables are the problem. See also "recommender systems", "info filtering systems", "decision-making systems", "credit scoring". Predictive models are, increasingly, the tools we will be relying on to run our institutions, deploy our resources, and manage our lives. But as I’ve tried to show throughout this book, these models are constructed not just from data but from the choices we make about which data to pay attention to—and which to leave out. Those choices are not just about logistics, profits, and efficiency. They are fundamentally moral... Big Data processes codify the past. They do not invent the future. Doing that requires moral imagination, and that’s something only humans can provide. We have to explicitly embed better values into our algorithms, creating models that follow our ethical lead. --- She covers most of the objections I would make to a less subtle author: It is true, as data boosters are quick to point out, that the human brain runs internal models of its own, and they’re often tinged with prejudice or self-interest. So its outputs—in this case, teacher evaluations—must also be audited for fairness. And these audits have to be carefully designed and tested by human beings, and afterward automated. In the meantime, mathematicians can get to work on devising models to help teachers measure their own effectiveness and improve. She does not include inaccuracy as a named criterion for WMDs, but her discussions sometimes require it. This is maybe the core shortcoming of the book: it doesn't wrestle much with the hard tradeoff involved in when modelling unfair situations, e.g. living in a bad neighbourhood which increases your risks and insurance costs through no fault of your own. She comes down straightforwardly on the direct "make the model pretend it isn't there" diktat. But then she notes a case where fairness trumped accuracy and still sucked. "Value-added modelling" of teacher quality: The teacher scores derived from the tests measured nothing. This may sound like hyperbole. After all, kids took tests, and those scores contributed to Clifford’s. That much is true. But Clifford’s scores, both his humiliating 6 and his chest-thumping 96, were based almost entirely on approximations that were so weak they were essentially random. The problem was that the administrators lost track of accuracy in their quest to be fair. They understood that it wasn’t right for teachers in rich schools to get too much credit when the sons and daughters of doctors and lawyers marched off toward elite universities. Nor should teachers in poor districts be held to the same standards of achievement. We cannot expect them to perform 1miracles. So instead of measuring teachers on an absolute scale, they tried to adjust for social inequalities in the model. Instead of comparing Tim Clifford’s students to others in different neighborhoods, they would compare them with forecast models of themselves. The students each had a predicted score. If they surpassed this prediction, the teacher got the credit. If they came up short, the teacher got the blame. If that sounds primitive to you, believe me, it is. My preferred measure would be to not prevent models from being rational, but instead make transfers to the victims of empirically unfair situation. (This looks pointlessly indirect, but price theory, and the harms of messing with them, is one of the few replicated economic.) My measure has the advantage of not requiring a massive interpretative creep of regulation: you just see what the models do as black boxes and then levy justice taxes after. Statistically speaking, in these attempts to free the tests from class and color, the administrators moved from a primary to a secondary model. Instead of basing scores on direct measurement of the students, they based them on the so-called error term — the gap between results and expectations. Mathematically, this is a much sketchier proposition. Since the expectations themselves are derived from statistics, these amount to guesses on top of guesses. The result is a model with loads of random results, what statisticians call “noise.” --- * Standardisation - the agreement of units and interfaces that everyone can use - is one of those boring but absolutely vital and inspiring human achievements. WMDs are a dark standardisation. * In her account, a model doesn't have to be predatory to be a WMD: for instance the recidivism estimator was first formulated by a public-spirited gent. * She says "efficiency" when she often means "accuracy, and thereby efficiency". This makes sense rhetorically, because she doesn't want to give the predatory models a halo effect from the less backhanded word "accurate". Also lards the texts with "Big Data" * The writer Charles Stross, asked recently to write the shortest possible horror story, responded: "Permanent Transferrable Employee Record". O'Neil makes us consider that this could be easily augmented with "including employee medical histories". * Surprisingly big evidential gaps: By 2009, it was clear that the lessons of the market collapse had brought no new direction to the world of finance and had instilled no new values. The lobbyists succeeded, for the most part, and the game remained the same: to rope in dumb money. Except for a few regulations that added a few hoops to jump through, life went on. * The car crash of American higher education is pretty engrossing. She credits the US News ranking with creating the whole mess though, which can't be right. Certainly it had some effect, and could fix some of its harm by including tuition fee size as a negative factor. even those who claw their way into a top college lose out. If you think about it, the college admissions game, while lucrative for some, has virtually no educational value. The complex and fraught production simply re-sorts and reranks the very same pool of eighteen-year-old kids in newfangled ways. They don’t master important skills by jumping through many more hoops or writing meticulously targeted college essays under the watchful eye of professional tutors. Others scrounge online for cut-rate versions of those tutors. All of them, from the rich to the working class, are simply being trained to fit into an enormous machine—to satisfy a WMD. And at the end of the ordeal, many of them will be saddled with debt that will take decades to pay off. They’re pawns in an arms race, and it’s a particularly nasty one. Anywhere you find the combination of great need and ignorance, you’ll likely see predatory ads. All of these data points were proxies. In his search for financial responsibility, the banker could have dispassionately studied the numbers (as some exemplary bankers no doubt did). But instead he drew correlations to race, religion, and family connections. In doing so, he avoided scrutinizing the borrower as an individual and instead placed him in a group of people — what statisticians today would call a “bucket.” “People like you,” he decided, could or could not be trusted. Fair and Isaac’s great advance was to ditch the proxies in favor of the relevant financial data, like past behavior with respect to paying bills. They focused their analysis on the individual in question—and not on other people with similar attributes. E-scores, by contrast, march us back in time. They analyze the individual through a veritable blizzard of proxies. In a few milliseconds, they carry out thousands of “people like you” calculations. And if enough of these “similar” people turn out to be deadbeats or, worse, criminals, that individual will be treated accordingly. From time to time, people ask me how to teach ethics to a class of data scientists. I usually begin with a discussion of how to build an e-score model and ask them whether it makes sense to use “race” as an input in the model. They inevitably respond that such a question would be unfair and probably illegal. The next question is whether to use “zip code.” This seems fair enough, at first. But it doesn’t take long for the students to see that they are codifying past injustices into their model. When they include an attribute such as “zip code,” they are expressing the opinion that the history of human behavior in that patch of real estate should determine, at least in part, what kind of loan a person who lives there should get. In other words, the modelers for e-scores have to make do with trying to answer the question “How have people like you behaved in the past?” when ideally they would ask, “How have you behaved in the past?” The difference between these two questions is vast. Imagine if a highly motivated and responsible person with modest immigrant beginnings is trying to start a business and needs to rely on such a system for early investment. Who would take a chance on such a person? Probably not a model trained on such demographic and behavioral data. should note that in the statistical universe proxies inhabit, they often work. More times than not, birds of a feather do fly together. Rich people buy cruises and BMWs. All too often, poor people need a payday loan. And since these statistical models appear to work much of the time, efficiency rises and profits surge. Investors double down on scientific systems that can place thousands of people into what appear to be the correct buckets. It’s the triumph of Big Data. Microcosm: This is not to say that personnel departments across America are intentionally building a poverty trap, much less a racist one. They no doubt believe that credit reports hold relevant facts that help them make important decisions. After all, “The more data, the better” is the guiding principle of the Information Age. Yet in the name of fairness, some of this data should remain uncrunched. --- Occasional data-poor hyperbole: Insurance is an industry that draws on the majority of the community to respond to the needs of an unfortunate minority. In the villages we lived in centuries ago, families, religious groups, and neighbors helped look after each other when fire, accident, or illness struck. In the market economy, we outsource this care to insurance companies, which keep a portion of the money for themselves and call it profit. Mistaken in the US: the "loss ratio" of US insurance as a whole is greater than 100: loss-making except for financial return on held premiums. Workers often don’t have a clue about when they’ll be called to work. They are summoned by an arbitrary program. Scheduling software also creates a poisonous feedback loop. Consider Jannette Navarro. Her haphazard scheduling made it impossible for her to return to school, which dampened her employment prospects and kept her in the oversupplied pool of low-wage workers. The long and irregular hours also make it hard for workers to organize or to protest for better conditions. Instead, they face heightened anxiety and sleep deprivation, which causes dramatic mood swings and is responsible for an estimated 13 percent of highway deaths. Worse yet, since the software is designed to save companies money, it often limits workers’ hours to fewer than thirty per week, so that they are not eligible for company health insurance. And with their chaotic schedules, most find it impossible to make time for a second job. It’s almost as if the software were designed expressly to punish low-wage workers and to keep them down. The solution for the statisticians at St. George’s—and for those in other industries—would be to build a digital version of a blind audition eliminating proxies such as geography, gender, race, or name to focus only on data relevant to medical education. The key is to analyze the skills each candidate brings to the school, not to judge him or her by comparison with people who seem similar... we’ve seen time and again that mathematical models can sift through data to locate people who are likely to face great challenges, whether from crime, poverty, or education. It’s up to society whether to use that intelligence to reject and punish them—or to reach out to them with the resources they need. We can use the scale and efficiency that make WMDs so pernicious in order to help people. It all depends on the objective we choose. But how do know we what's relevant to medical education, except by correlation discovery? It would also be a cinch to pump up the income numbers for graduates. All colleges would have to do is shrink their liberal arts programs, and get rid of education departments and social work departments while they’re at it, since teachers and social workers make less money than engineers, chemists, and computer scientists. But they’re no less valuable to society. This is obvious true on average. But on the margin? --- A 'Weapon of Math Destruction' is a model which is unaccountably damaging to many people's lives. But she doesn't cash out her criteria. So I did it for her. When is a system dangerous?: Opacity Is the subject aware they are being modelled? Is the subject aware of the model's outputs? Is the subject aware of the model's predictors and weights? Is the data the model uses open? Is it dynamic - does it update on its failed predictions? Scale Does the model make decisions about many thousands of people? Is the model famous enough to change incentives in its domain? Does the model cause vicious feedback loops? Does the model assign high-variance population estimates to individuals? Damage Does the model work against the subject's interests? If yes, does the model do so in the social interest? Is the model fully automated, i.e. does it make decisions as well as predictions? Does the model take into account things it shouldn't? Do its false positives do harm? Do its true positives? Is the harm of a false positive symmetric with the good of a true positive? [Data #2, Theory #1, Theory #3, Values #1]
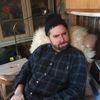
Digitisation has become a mantra and today it seems like everything has to be replaced by a digital solution and in this particular case systems. This in itself is stupid and unintelligent in my opinion. The book opened my eyes to a world of bias, unfair and and manipulating systems that are already impacting a lot of peoples lives. what I think we have to remember is that these systems are build by people. Technologi is not bad in itself - it's how people or businesses utilise technology, whether it's for profit or power, that is despicable. I like that it's more than opinions - it has several cases from the US and know that I'm aware of the fact I hope I've become enlightened enough to spot these systems in Denmark where I'm from.
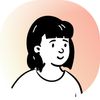
The book provides great examples and a way of identifying and framing these algorithmic problems.
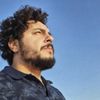
This is just another book that proves that all those smart-ass software applications should not be left unattainable by human supervision and revision.
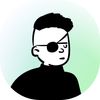
This book is more about its subtitle than its title. Lots of ranting about injustice and lots of presumptions that mathematical models are a prime mover of it. If your only tool is a hammer, lots of things start looking like a nail. The author is a mathematician, but I don't think you can lay all of these problems at the feet of mathematical models. People and institutions are greedy and lazy, and always have been. For profit colleges are able to rip people off for lots of reasons, not just "predatory ads" targeted by mathematical models. She talks about policing and models that target high crime areas. There are lots of interesting and difficult issues related to that but all she really does is rail against stop and frisk policies. I think people and institutions misuse data, analysis and mathematical models all the time, but this book didn't really get into that in any depth.
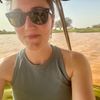
So I think this subject is fascinating, first of all. And I might be biased because I’ve read many articles and reports and listened to lots of podcasts on this exact topic. BUT nonetheless, the book feels a bit obvious/surface level. Good points, definitely, but not the deep dive I expected. 🤷🏻♀️
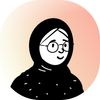
It's an important book for this day and age because Cathy O'Neil makes a complex issue both accessible and comprehensible without dumbing it down. As a way for people to get into the topic, it's excellent and deserves all the attention it currently gets. But it's not something people who are engaged in this topic necessarily need to read. And it's too focused on US examples.
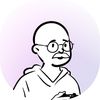
I had much higher hopes for this book. While I enjoyed it, I had hoped for a more comprehensive narrative instead of a piece by piece look at the various "WMD"s. Admittedly, it has been over a week since I finished the book so my review comes less than fresh. However, the above is my lasting impression of this book.
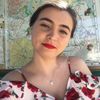
Loved this book- it was a class assignment and I learned a lot about Big Data.
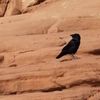
Yes, this book does deserve its title in all caps. Written by Cathy O’Neal, who loves numbers possibly more than I do, this book does an excellent job of showing the public all of the algorithms that rule our lives that aren’t ideal and why they aren’t ideal. In a non-mathy way, which makes me quite sad and why I have rated this book 4/5. I wanted my math! Back to the book. What makes this book great is O’Neal doesn’t just talk about the worst WMDs (Weapons of Math Destruction – and remember that, because that is how she refers to them) but also covers some systems that DO work really well and WHY they work. If you are frustrated with the outcomes that keep coming up in your life, read this book. If you hate math but want to understand what is going on with all those ads, how what you do can affect so many different things throughout you life! Well written and easy to read, this book was flowing and well paced. A good read, I don’t want to spoil this for anyone interested. It’s worth the read and no single part is better than the rest. If you are interested in what controls so many things around you, read this. Read this now. I received this book from Blogging for Books for this review.
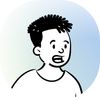
Underwhelmed. Too US-focused, sometimes seriously outdated, not much value for someone already experienced in the field. On the other side it’s a good 101-level intro to unintended consequences of big data algorithms applied to various aspects of human lives.
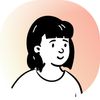
I thought that this book was great, and a little alarming. It's a book that needs to be read, since algorithms are controlling an ever-increasing portion of our lives. The case studies in the book are interesting and I like that O'Neil doesn't take a soft stance on the failure of these models to help people. Like her, I love mathematics, but these models can't be allowed to proliferate without any checks on them, as what seems to be the case at the moment. I found the author's writing to be engaging, and the message is important. Read this book.
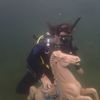
Very important book for the modern tech age. Deeply informed criticism. Influential, if rising calls for regulating tech giants bear out. I have been writing a great deal about the book for our book club. We're just past the half-way mark now. You can find my posts, other people's comments, and other folks' blog posts here.
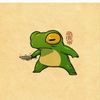
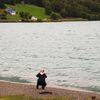
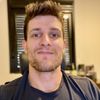
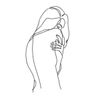
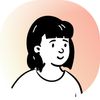
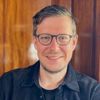
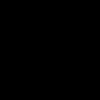
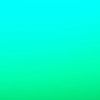